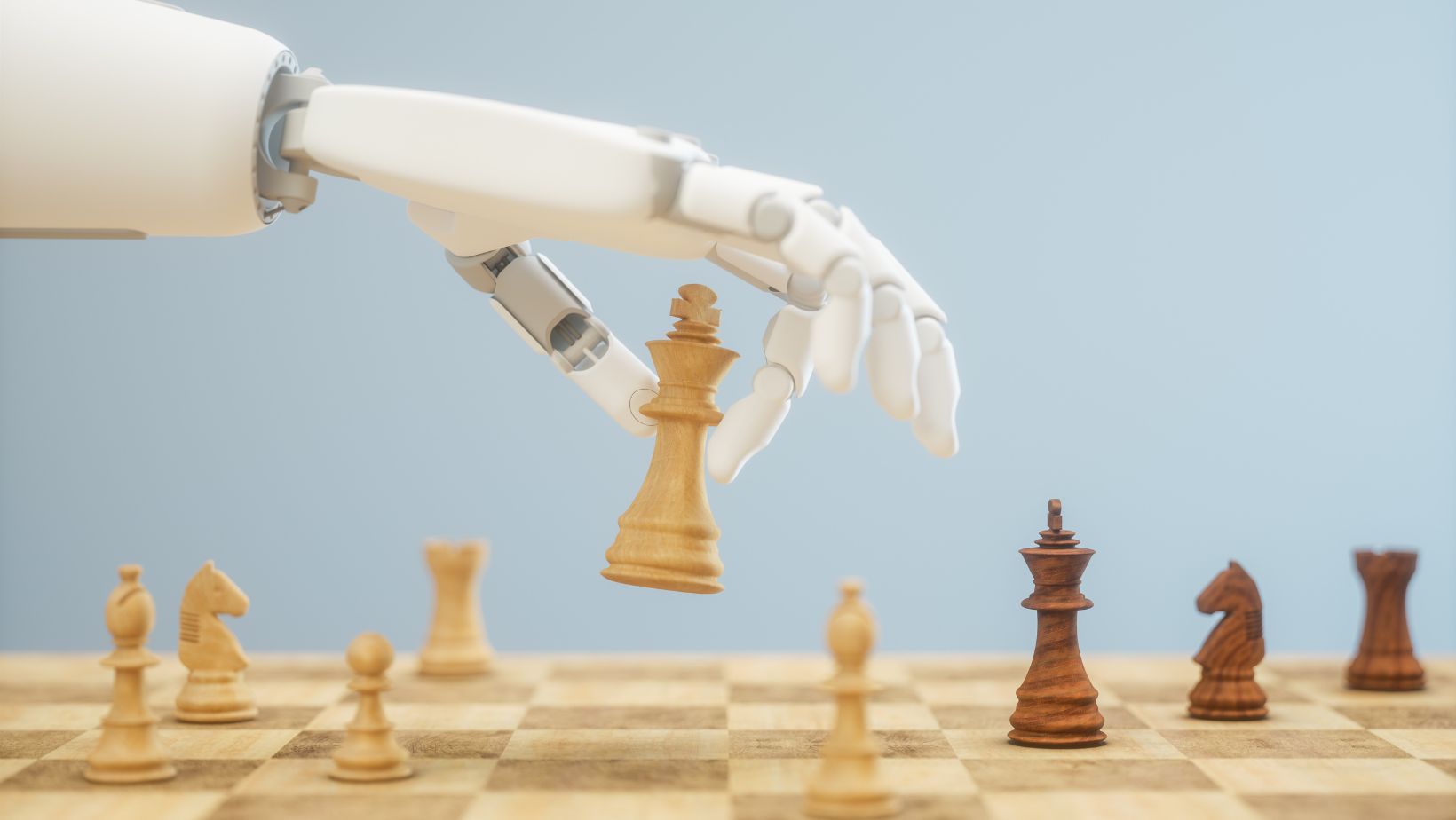
Where is the history of machine translation going? Part 2
Anyone who has even heard about new technologies coming out in the open may (or maybe should) have wondered about their downside. You don’t need to be a sci-fi aficionado, imagining tech dystopias ranging from HAL 9000[1] to the Borg Collective[2], to expect the worse.
Language Technology has its fair share of foreseeable disasters coming. Here is a brief overview of some that have already come (the order is casual and the list far from exhaustive):
Unethical Use
Unfortunately, we know that people are not always good to each other. This means that there are surely some people who will try to use AI to create harmful content.
An interesting example was Meta’s Galactica[3], that made up false papers attributing them to real authors, or the criminal use of some image generating systems (that usually include a part of language understanding, as you can describe the image you want to get in natural language) to create child pornography[4].
Bias
A very well-known issue with AI in general, which is also quite recognisable in neural machine translation as well, is the presence of bias in the output, ranging from gender[5] to racial[6].
Biases are errors produced as a consequence of a certain distribution of factors in the training data of a model. In other terms, if in the data you use to teach the model how to speak doctors are always males and baby-sitters are always female, the algorithm will not ever wonder if it can be the other way round and, in case of neural machine translation, it may even twist the translation to grammatically accommodate this world view.
Then again how would humans behave in this situation?
Environmental Concerns
AI is not cheap to produce, neither in economic terms nor in environmental ones. Without even taking into consideration LLMs[7], whose carbon emissions during training are roughly estimated as being equivalent to that of 550 8000-km-long trips, all neural networks, including the Transformers used in neural machine translation, have a considerable environmental footprint[8]. While the latter may be easier to estimate during the creation of the AI, that is to say during training of the models, it is extremely complicated to evaluate it during inference (that is, when people use it sending requests).
“Garbage out” of Black Boxes
While the expression “garbage in, garbage out” has long been known in the translation industry, meaning that the quality both of the training data and of the source text would inevitably influence the quality of the automatic translation, neural networks may have created a “Garbage out”-only problem.
These machines do not have what we call ‘common sense’ (though I agree that this concept is somewhat debatable even among humans…), which means that they do not know if what they are saying is logical or correct. This kind of mistakes can be due to incorrect information retrieved from the training data, but they can also be a so-called hallucination[9], a confident but wrong answer that does not seem to have an explanation in the original data.
The big issue here is that most neural networks are ‘black boxes’[10]: they are not able to explain how they got to the proposed conclusion, and we are not able to back-engineer where it all went south.
Data Privacy
You may have heard this before: if something is free, you are the product. Or, in this case, your data is.
I already mentioned that all AI models are trained on large amounts of data, and this data needs to come from somewhere.
While legislation worldwide struggles to keep up with the development of new technologies, it is fairly easy to find loopholes and scavenge for whatever it is needed within the tons of data that we carelessly put out there. Including most of the data we feed to AIs to get the answers we want.
Have you ever tried chatting in front of Alexa about buying a new pair of slippers and then being flooded with shoe shops advertisement?
Remember that Alexa is based on an LLM, and he/she/it/they is listening[11]… (though the producers say otherwise[12].)
Data ownership
Once said data has been collected, who owns it?
Do translators still own the translation when it was delivered to the client? Even if it is published on the web?
And if the translated text is then used to train a machine translation engine, should the translator (and/or the owner of the original text) be granted a fee?
All good questions for which we do not currently have an answer to, as the technology is so new and evolving so rapidly that global laws are still lagging behind.
(Although, if I had a say in this, I’d remind you of a previous article published on this blog[13], where I discussed the way machines ‘see’ the words they are fed. Considering the representation and segmentation they apply to ‘read’, I personally doubt that the text that they receive can be considered as part of the original copyright.)
Accountability
There is also another legal hurdle to overcome. Who is responsible for AI?
If a medical translation by a machine resulted in harm to the patient, who should be held responsible? We could blame the software developer for creating a faulty machine, the doctors for not checking the translation, the AI itself for the output it produced.
And conversely, should AI creations result in copyright attribution? And if so, should the model own the rights to its work[14]?
Labor Exploitation
When we think of artificial intelligence, including machine translation, we usually think of top researchers, highly paid corporate workers, and automatic processes.
Alas, the truth is that today AI-related jobs are often underpaid, alienating, repetitive, with cut-throat turnaround times and competition practices, outsourced and crowdsourced[15].
On the backend, swarms of people are involved in tasks like data annotation (a process to prepare data for use in the AI training), toxic content moderation[16], and data gathering.
On the user-end, AI based solutions (again including neural machine translation, as well as LLMs) are being used to reduce rates for vendors, shorten delivery times, lower quality expectations (though this may be unintentional and not monitored), and making life generally harder for people who have to work with models’ outputs.
Job Replacement
Almost anyone working in the field will tell you that “AI is not going to take away people’s jobs.”
I argue that this may be true in general, as AI will not leave humans jobless. But it may take away THAT job. The one that people liked to do, that have studied to do and which they were good at. AI may turn that job into something else.
Translators may not be unemployed in the future, but will they still be translators, or will they need to become something different? Should they switch to being post-editors, programmers, field experts (whatever this is), data curators?
And do they want to? Do they have a choice?
And should they have a choice?
[1] 2001: A Space Odyssey – Wikipedia
[2] Star Trek’s Borg – Wikipedia
[3] Why Meta’s latest large language model survived only three days online – MIT Technology Review
[4] AI-generated child sex images spawn new nightmare for the web – The Washington Post
[5] She is pretty, he is smart: More on gender bias in MT with Dr. Eva Vanmassenhove – machinetranslation.com
[6] Microsoft shuts down AI chatbot after it turned into a Nazi – CBS News – Tay is a dated example of how quickly things can go wrong.
[7] The Generative AI Race Has a Dirty Secret – Wired
[8] Sterionov D., Vanmassenhove E. – The Ecological Footprint of Neural Machine Translation Systems
[9] What is a translation hallucination and how do I deal with them? – Unbabel Support
[10] Opening the ‘black box’ of artificial intelligence – Horizon (EU commission)
[11] Amazon’s Alexa Never Stops Listening to You. Should You Worry? – Wirecutter (NYT)
[12] How Alexa works: Wake word – Amazon.com
[13] Do You See What I See? – Human Parity in Translation – Aglatech14 Blog
[14] Artificial Intelligence and Legal Personality – Liedekerke – The article does not take into account a third party which is heavily involved in particular kinds of AI such as LLMs, i.e. the user creating the prompts.
[15] The Exploited Labor Behind Artificial Intelligence – Noema
[16] OpenAI Used Kenyan Workers on Less Than $2 Per Hour to Make ChatGPT Less Toxic – TIME
Elena Murgolo – Language Technology R&D Lead Orbital14
Elena started out as a conference interpreter for English, German and Italian, but grew attached to machines and ended up combining the two worlds by specialising in translation software, machine translation and language technology in general. In recent years, she has presented at various international conferences (MT Summit, NETTT, EAMT) and tried to pass on her passion through courses in specialised master courses (EM TTI). Her papers include: Murgolo E., Productivity Evaluation in MT Post-Editing and Fuzzy Matches Editing. Setting the Threshold; Murgolo E. et al., A Quality Estimation and Quality Evaluation Tool for the Translation Industry. However, to satisfy her evil side as well, she also reviews the papers of other experts as a member of the Programme Committees of the same conferences she speaks at (TRITON, Hit-IT, NLP4TIA).
Elena nasce come interprete di conferenza per inglese, tedesco e italiano, ma si affeziona alle macchine e finisce per unire i due mondi specializzandosi nella gestione dei software di traduzione, nella traduzione automatica e nella tecnologia linguistica in genere. Negli ultimi anni presenta in diverse conferenze internazionali (MT Summit, NETTT, EAMT) e cerca di trasmettere la sua passione attraverso corsi in master specialistici (EM TTI). Tra i suoi paper: Murgolo E., Productivity Evaluation in MT Post-Editing and Fuzzy Matches Editing. Setting the Threshold; Murgolo E. et al., A Quality Estimation and Quality Evaluation Tool for the Translation Industry. Per soddisfare però anche una certa innata cattiveria, rivede i paper di altri esperti come membro di alcuni Programme Commitee delle stesse conferenze in cui presenta (TRITON, Hit-IT, NLP4TIA).